Abstract
Learning while sleeping is a long-standing human fantasy, although studies have revealed that humans are not aware of what transpires when sleeping. Nevertheless, an absence of conscious knowledge fails to rule out the premise that individuals discern and recollect occurrences when asleep. The distinct functions involved in the retention of memories are currently being studied at multiple tiers, spanning from molecular reactions to whole-brain engagement. The Brain-Computer Interface (BCI) technique is a potent tool for user-system connection. BCI technologies are implemented to give impaired persons recourse to the information offered through standard functions. This review aims to determine whether using BCI technology to facilitate learning in sleep is practical and effective. The study will examine how BCI may stimulate particular brain activity during sleep, ease recollection and consolidation, and encourage learning new concepts. A multidisciplinary approach will be used in the investigation, integrating electroencephalography (EEG) recordings with behavioural and subjective indicators of learning performance. The findings of this study will shed light on how BCI technology may be used to improve learning and cognitive function when a person is sleeping, having implications for educational settings and cognitive therapy.
Keywords: brain-computer interfaces, BCI, learning, sleep, memory consolidation, neural stimulation, cognitive performance. Top of Form
Sleep is a rapid-transitional condition that comprises a lack of awareness, reduced action, and the capacity to react to external cues. It is crucial for learning procedures as it helps consolidate memories (Wang, 2020). Although memory consolidation is not the sole objective of sleep, it serves the most fundamental function as it contributes to establishing cognition in alertness (Puchkova, 2020). The fluidity of memory is solely established when the mind’s innate recollections are perpetually referred to and associated with the present-moment emotions. Since several academics and psychologists argue that memory is a crucial component of the mental state, it would be thrilling to discover that memory is created and developed substantially while asleep (Born & Wilhelm, 2011). In the neural structures of the human brain, awareness and the creation of persistent memory constitute distinct phenomena deemed incompatible and unable to coincide. Therefore, memory building could represent a sleep component that ultimately enables individuals to comprehend how sleeping is connected to a varying degree of cognitive decline.
However, specific research findings indicate that daily occurrences are neither recalled nor acknowledged on subsequent days (Ruch & Henke, 2020). These outcomes support the idea that sleep may not be the optimal period for lasting memory development due to changes within the brain functions, genetic expression trends, and synaptic remodelling. However, several of such issues fluctuate throughout sleep. Since knowledge obtained while awake is eventually reinforced by neural repetition while sleeping to assess the mental process in sleep, certain structural modifications to memory structures occur when sleeping. According to Rasch & Born (2013), every living can preserve and regain memories, which allows action modification in response to the circumstances of a dynamic setting and to determine and cultivate habits in a specific collection properly.
Learning is among the most efficient neurological processes, and besides promoting cognitive and interpersonal growth, it also empowers individuals to pursue professions that advance a particular standard of living. Innovative developments have emerged from addressing the disparity in interactions between individuals and computers utilizing various technologies. Brain-Computer Interface (BCI) is among the emerging techniques in the field of neurology that enable direct brain-to-external device connection via conventional neuromuscular avenues (Mudgal et al., 2020). The brain-computer interface (BCI) infrastructure takes the brain’s messages and evaluates transfers, and converts them to instructions which are subsequently sent to programs that carry out specific activities, enabling direct brain-to-external-device connection (Camargo-Vargas et al., 2021) (Fig 1.1). BCI interfaces translate neural activity effectively into management and interaction for people with erratic or inadequate motor abilities. The programs enable the brain to communicate fluidly with an electronic system, attracting significant interest in areas like artificial intelligence (Abdulkader et al., 2015). Innovation for brain-computer interfaces (BCI) holds the prospect of improving the academic setting that confronts numerous obstacles and inefficiencies.
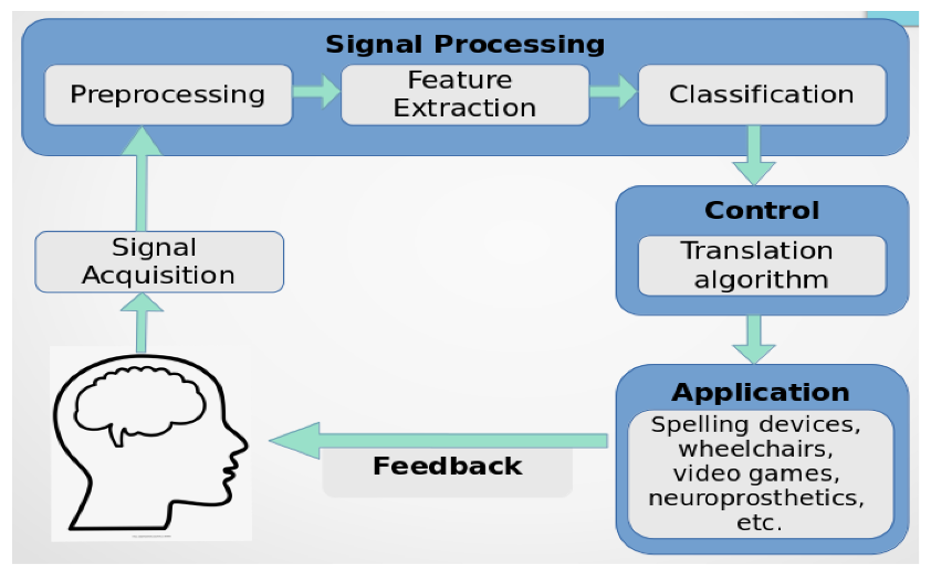
An in-depth comprehension of neural processes can be provided through cognitive and emotional BCIs that could enhance learning techniques and boost brain-associated abilities. They can provide a more robust scientific basis for learning and instruction procedures, such as modifying instructional material to correlate with brain functions, gauging students’ engagement in a particular subject, and assisting them in focusing on particular assignments (Jamil et al., 2021). In addition, the electroencephalogram (EEG) provides an alternative physiological method for evaluating learners’ cognitive effort in a learning environment (Zhu et al., 2021). By capturing the electrical impulses generated by neurons in a real-world setting, it can instantly examine one’s mental condition. The EEG-BCI examines a bi-directional connection involving the brain and foreign equipment. Individuals can obtain several distinct spectrum responses by contacting the EEG sensors inside the brain’s cortical location (Cao, 2020) (Fig 1.2 and 1.3). The number of intellectual assets needed to complete an activity is represented by mental energy. It is viewed as a synthesis of the features of the alleged request, the complexity of the computation of data, and individual competence. When there is little contact between variables, task-related data could be utilized in the learning component at once as there are few connections between each component. Contrarily, highly interconnected data comprises components that strongly link to one another and must be merged with extra details simultaneously.
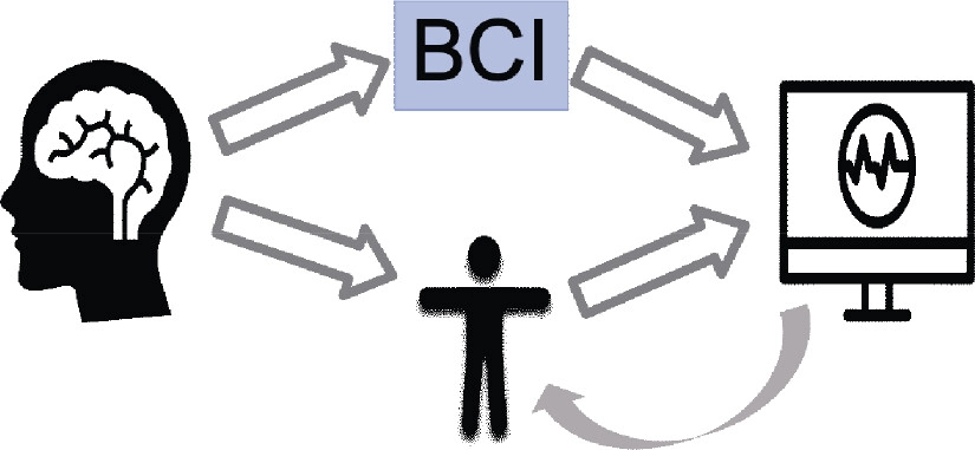
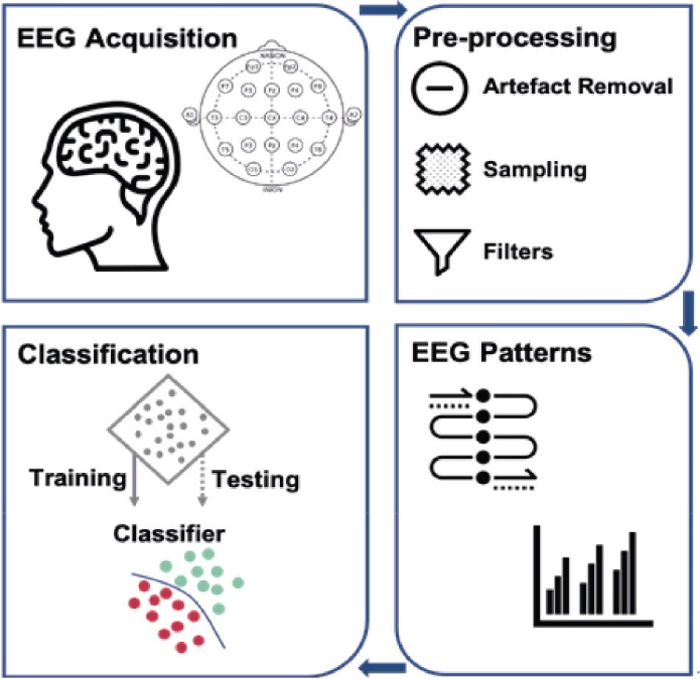
Literature Review
Knowledge and Memories during Sleep
Sleep is a complex physiological state in most animals, including humans. It is a fundamental process for preserving various biological procedures, including brain functions like memory and learning. Sleep supports several fundamental physiological processes, including immune function, modulation of neurotransmitters, and reconstruction of tissues. The heart rate, blood sugar control, insulin response, and hypertension all rise due to inadequate Sleep (Sevasti Kapsi et al., 2020). Additionally, it can cause growth-related, mental, and psychological conditions. Most sensations get inactive during sleep, making one less responsive to their surroundings. Visual interaction vanishes, the human body is essentially still, and the response to sound is scrambled. This channel linking long-term cognition stops when short-term cognition ceases relaying data. Memories of past events may be kept up to three minutes before they dissipate when someone falls asleep.
Numerous research has been conducted to explore how sleep affects learning and cognition in light of the long-established significance of sleep for cognitive functions. Research by Pierre Maquet (2001) reviews present-time knowledge on the role of sleep in cognitive processes, with a particular emphasis on learning and memory. Maquet (2001) begins with a brief discussion of the historical perspectives on the link between cognition and sleep. He notes that many of the early studies on this topic were conducted on animals and that they suggested a direct connection between memory retention and sleep consolidation. He then turns to more recent research on humans, which has provided additional knowledge on the foundational connection.
According to Maquet, distinct unique sleep phases—slow-wave Sleep (SWS) and rapid eye movement (REM) Sleep—are significant for consolidating memories. Although REM sleep is defined by rapid, erratic neural activity and quick eye blinks, slow, synchronized brain waves accompany SWS. Maquet suggests that SWS is essential for consolidating declarative memories, which are memories of facts and events. In addition, Maquet notes that during SWS, the brain undergoes a process of replaying memories, which involves resetting active brain pathways when the memories are being encoded. This replaying process is thought to strengthen the connections between neurons, thus enhancing memory consolidation. However, while an individual is in a REM state of sleep, the brain may undergo a process of unlearning, eliminating unnecessary or outdated information. Maquet notes that while much remains to be learned about the unique relationship, the evidence suggests that sleeping is essential for forming fresh recollections. He also suggests that further research may shed light on the mechanisms underlying this relationship and lead to new approaches for enhancing memory consolidation.
However, there are a few limitations to the research by Maquet (2001) worth noting. Firstly, while Maquet provides a clear summary of the existing evidence, he needs to provide a more critical analysis of this evidence. He needs to discuss potential confounds or limitations of some of the studies he cites. Secondly, Maquet’s research was published over twenty years ago, and there have been many new studies on the topic since then. Therefore, while his research is an excellent starting point for understanding the role in question, it should be supplemented with more recent research. Since its publishing, there has been much new research on the brain-sleep connection (Nemeth et al., 2021). One central area of focus has been on the neural mechanisms underlying this relationship. For example, a study by Prehn-Kristensen et al. (2014) used transcranial oscillating direct current stimulation (DCS) to enhance slow-wave oscillations during sleep, which led to improved memory consolidation. That suggests that the slow-wave activity during SWS may be a critical factor in memory consolidation. This research is further supported by Vulić et al. (2021), who argue that several elements of knowledge can be remembered and retrieved via associative memory (AM). The data connecting mechanism at the heart of AM enables the subsequent recollection of how particular traits or bits of data relate and co-exist. The associative regions of the region of parietal and other regions, together with their aligned connections to the hippocampus, additionally serve a crucial part in these procedures. However, the hippocampus and other regions within the medial temporal are generally related to linking and associative memory.
In addition, recent research (Cousins & Fernández, 2019) has examined how sleep deprivation affects memory and found that it may impede the recollection of declarative memories if the sleep deprivation occurs during the early hours of the morning when SWS is most prominent. That supports Maquet’s suggestion that SWS is particularly important for consolidating declarative memories. In addition, a study by (Payne et al., 2012) found that sleep can facilitate the formation of emotional memories for negative emotional stimuli. That implies that integrating and digesting emotional information may be influenced by sleep.
Recent studies have explored potential interventions for increasing memory consolidation while sleeping and exploring the mental functions underlying the association between memories and sleeping. Neumann et al. (2020) discovered that the odor markers provided before learning and during SWS could enhance memory consolidation. That suggests that external stimuli presented during sleep may be able to modulate the consolidation of memories. Another area of research by (Guo et al., 2013) has examined how sleep disorders impacted the consolidation of memories and discovered that patients with obstructive sleep apnea have impaired memory consolidation, particularly for declarative memory. Individuals experiencing respiratory problems during sleep have reduced sleep quality and varying degrees of memory impairment. That suggests that sleep disorders may harm cognitive processes.
A Molecular Perspective by Brito (2021) extensively examines the genetic concepts behind memory retention procedures and sleep’s function in such processes. Furthermore, (van & Walker, 2011) focuses on The hyperlink between emotional recollection and sleeping, while Cristiane Furini et al. (2020) provide a detailed analysis of the chemical processes that contribute to the retention of memories in the hippocampus, which is a brain structure involved in memory formation. The research highlights the role of genetic production and synthesis of proteins during the fusion of memories and how these processes are regulated during sleep. The authors propose that sleep helps to consolidate memories by promoting the synthesis of proteins involved in memory synthesis.
Furthermore, a study by Reyes-Resina et al. (2021) suggests that molecular consolidation is regulated by genes and proteins activated during sleep. It is further supported by Wang et al. (2018), who highlight how CREB (cAMP response element-binding protein), an encoding component, plays a role in the retention of memories, which is activated during sleep. This protein significantly impacts synthesizing proteins involved in memory consolidation, such as BDNF (brain-derived neurotrophic factor), which promotes brain neurons’ growth and survival (Wang et al., 2018). In addition, Cunningham et al. (2014) provide insights into dynamic memory synthesis. The authors suggest that the development of psychological recollections is greatly aided by REM sleep by enhancing the emotional salience of these memories. The research highlights the part played by the amygdala, a brain region responsible for emotional processing, in consolidating emotional memories during REM sleep. They propose that the amygdala stimulation following REM sleep helps to consolidate emotional memories by enhancing their emotional intensity, which aids in the consolidation process.
The Ability to Learn During Sleep
Learning during sleep has been a concern for researchers in sleep science and cognition for several decades (Poe et al., 2010). The capacity to acquire new knowledge during sleep is a fascinating study area as it can enhance learning and memory consolidation in individuals. Recent research by (Colten et al., 2023) investigates how individuals may gain new concepts while asleep. According to, (Colten et al., 2023), Non-REM (NREM) sleep and REM sleep are the major sleep phases. Slow neural processes are a hallmark of NREM sleep, whereas REM sleep is rapid eyesight shifts and vivid dreaming. Sleep has been linked to memory consolidation, following many studies showing that enough sleep is essential in synthesizing declarative and procedural memory (Born & Wilhelm, 2011); (Stickgold & Walker, 2007). Memory consolidation is a process that involves relocating memories from temporary to permanent storage. Studies have shown that sleep enhances memory consolidation by promoting the replay of newly acquired memories during sleep, leading to long-term memory consolidation (Stickgold & Walker, 2007). According to (Reyes-Resina et al., 2021), memory rehashing during sleep is facilitated by a neuron’s ability to become functional soon after learning, hence allowing the enhancement of associations between neurons and the formation of new synaptic connections.
Recent studies have suggested that sleep consolidates memories and facilitates learning new information. (Stickgold & Walker, 2007); (Navarrete & Lewis, 2019) confirmed that individuals could learn new knowledge when sleeping, presenting auditory stimuli to sleeping participants and observing the effects on memory consolidation. Comparing participants subjected to audio stimuli while they slept as opposed to those in the control category, the researchers discovered that those subjected to auditory cues while sleeping had improved memory retention for the information presented. The study (Puchkova, 2020) builds on earlier research that showed the potential for learning during sleep. Transdisciplinary studies by (Batterink et al., 2016); (and Pearson & Wilbiks, 2021) demonstrated that humans could learn to associate auditory cues with particular objects during sleep. Participants were presented with auditory cues that were combined with images of items. The investigators observed that individuals could recognize the objects when presented with the auditory cues during subsequent wakefulness, indicating that learning had occurred during sleep.
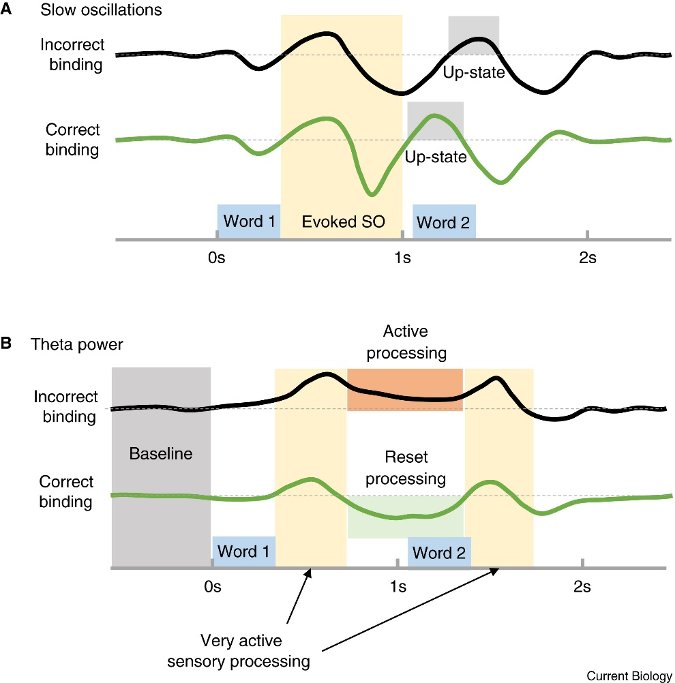
According to Navarrete and Lewis (2019) (Fig 1.4), the stimulation exposure length must fulfill two requirements for language acquisition to be implicitly encoded.
A – Initially, the second phrase to be encrypted has to occur within the up-states of the gradual waves, where the refining of words is encouraged by settings similar to a cerebral wake-up.
B – In the second requirement, decreased theta oscillation creates a period that permits phrase encoding. The reorganization of cortical neural pathways makes the brain further open to new stimulation, which increases the likelihood of encoding.
Different studies by (Andrillon & Kouider, 2016); (and Ruch et al., 2022) demonstrated that Individuals might acquire unfamiliar words while sleeping. Participants were exposed to unfamiliar phrases while they slept, and their capacity to remember them after awakening was assessed. Compared to the controls that were not given any stimulation, the researchers discovered that those subjected to unfamiliar phrases. At the same time, they slept and were more inclined to recall them. Animals have also been shown to be capable of learning while asleep, as the study by (Hall et al., 2015) demonstrated that dogs could learn to associate odors with specific behaviors during sleep. The researchers presented dogs with a specific odor and a subsequent treat when they performed a specific behavior. The smell was subsequently spread to the dogs during sleep, and the researchers observed that they performed the associated behavior upon waking.
An expanding collection of evidence indicates that sleep is essential for the synthesis of a variety of memories, including declarative memory (Alger et al., 2014), procedural memory (Rasch & Born, 2013), and emotional memory (Cunningham & Payne, 2017). The synthesis during sleep is believed to occur through the stimulation and strengthening of neural networks involved when knowledge is first encoded (Diekelmann & Born, 2010). The integration of language knowledge, particularly for younger children, is also thought to be affected by sleep. For instance, (Axelsson et al., 2016) discovered that participants who fell asleep upon learning a new vocabulary list performed better on a recall test than those who remained awake. Similarly, research by Schimke et al. (2021) demonstrated that sleep enhances the consolidation of new phrases in a non-native language. The present study adds to the corpus of knowledge by demonstrating the significance of sleep in adopting an innovative linguistic principle.
The current study is supported by Poe et al. (2010), who emphasize the significance of considering the timing and duration of sleep in the consolidation research. Moreover, Yngrid et al. (2022) asserted that participants who took a nap after learning a motor task did better on a retention test than those who slept later. The research further demonstrates that sleep immediately after learning is essential for consolidating a new linguistic rule. In addition, an investigation by Feld et al. (2016) found that participants who slept for many hours after learning a list of word pairs performed better on a recall test than those who slept for fewer hours. Therefore, more extended sleep could be essential in consolidating abstract knowledge, such as linguistic rules.
Language learning involves a complex interplay of cognitive and neural processes, including attention, working memory, and executive function Deldar et al. (2020). Prior investigations have proven that sleep can enhance these cognitive processes, facilitating language learning. For example, Tamminen et al. (2020) studied participants who slept after acquiring an additional language task and revealed increased activity in brain regions associated with attention and working memory compared to those who remained awake. There is evidence from multiple investigations that memory synthesis during sleep involves the reactivation and strengthening of neural networks involved in initial encoding (Dudai et al., 2015); (Paller & Voss, 2004); (Schechtman et al., 2023). According to studies, participants who slept after learning a visuospatial task showed increased activity in the hippocampus compared to those who remained awake.
The Application of BCI in Other Fields
Brain-Computer Interfaces (BCIs) are communication structures that convert neural information into physical movements, allowing individuals to interact with their environment without using traditional communication channels (Park, 2023). This technology has been utilized to enhance the standard of life for those with different neurological illnesses, including those with disorders of consciousness (DOCs) (Spataro et al., 2022). Disorders of consciousness are a collection of neurological illnesses characterized by altered states of awareness, including coma, an unconscious condition, and a vegetative instance (Edlow et al., 2020). Such conditions can result from traumatic brain injuries, strokes, and other neurological disorders. Patients with DOCs may have limited communication ability with their environment, leading to social isolation, decreased quality of life, and increased healthcare costs. Traditional methods of communication, such as speech and physical movement, may be impaired or absent in these patients, making it challenging to assess their cognitive function accurately.
BCIs have shown promise in providing a communication channel for patients with DOCs, enabling them to express their thoughts and interact with their environment. BCIs work by detecting and interpreting brain signals, including functional magnetic resonance imaging (fMRI), a magnetoencephalogram (MEG), and an electroencephalogram (EEG) (Dheeraj Rathee et al., 2021). The instructions created from these impulses can subsequently be used to operate other objects like computers, wheelchairs, and robotic arms. ECG and EOG sampling occur concurrently in MEG examinations as it facilitates cancellation and rectification of artifacts such as breathing, heartbeat, and eye blinking, among others, over the information preliminary processing stage (Fred et al., 2022) (Fig 1.5). Once the MEG signals are collected, their information is processed ahead of time to eliminate any unnecessary patterns not generated by the brain function.
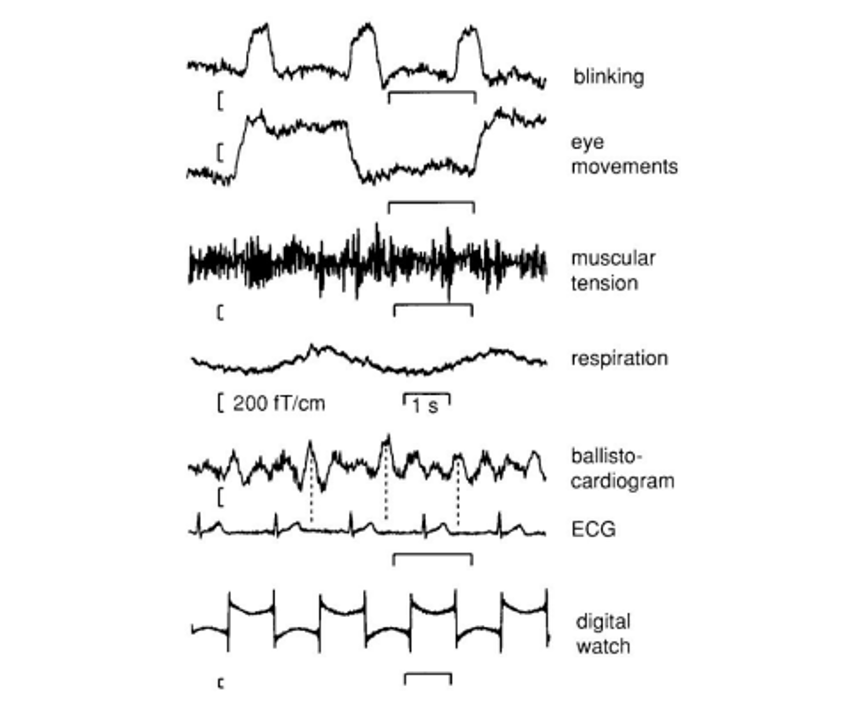
The use of BCIs in patients with DOCs has been the subject of numerous studies over the past decades (Mikołajewska & Mikołajewski, 2014). According to an analysis by Chaudhary et al. (2022), a patient with ALS who was in a locked-in state used a BCI to communicate with his family and medical team and could answer yes or no questions accurately, even composing sentences using the BCI. Another study by Gibson et al. (2016) demonstrated using a BCI to detect consciousness in patients with DOCs. The study found that some individuals with severe brain damage managed to interact with a BCI despite having a vegetative condition diagnosis. The researchers noted that the use of BCIs in patients with DOCs could potentially lead to improved patient outcomes, as it allows for a more accurate assessment of cognitive function and the potential for better communication with caregivers and family members.
Flesher et al. (2021) discovered that patients leveraging a BCI to operate a robotic arm succeeded while in a minimally conscious condition. The patient’s ability to pick up items and move them across a table showed how BCIs could enhance the standard of life of people with DOCs by enabling meaningful interactions with their surroundings. However, despite the promise of BCIs in patients with DOCs, several challenges still need to be addressed before the technology can be widely adopted in clinical practice (Valeriani et al., 2022); (Abdulkader et al., 2015). The absence of standardized BCI technology and technique is one of the primary issues. The generalizability of findings may be constrained. As a result, they needed to make it easier to compare results across investigations. BCIs’ price can also be a significant deterrent to being used in therapeutic settings, especially in situations with limited resources.
Classical Conditioning and Brain-State Classification
Classical conditioning, also known as Pavlovian conditioning, is an aspect of instruction where a stimulus that was initially impartial is combined with a signal that is uninfluenced to provoke a reaction in the absence of the unconditioned stimulus (Christoforou, 2017). In BCI research, classical conditioning can elicit specific brain responses utilizing electroencephalography to identify and categorize (EEG) or other brain imaging techniques (Ruf et al., 2013). For instance, a visual or auditory stimulus can be paired with a reward or punishment to elicit a specific brain response for event-related potential (ERP), which can be detected using EEG (Chikara et al., 2020). Brain-state classification is another technique used in BCI research that involves classifying EEG or other brain signals into different states based on activity patterns (Ilyas et al., 2016). This technique has been used to develop BCIs that allow users to modulate an individual’s neural function to operate third-party tools like computers or artificial limbs. However, Porsteinsson et al. (2021) assert that brain-state classification requires users to generate reliable and consistent brain signals, which may be difficult for AD patients with impaired cognitive function.
Classification for AD Patients
Several individuals globally have Alzheimer’s (AD), a devastating and chronic neurological illness (Kumar et al., 2022). Memory loss in people with AD can result in an inability to communicate effectively, leading to a decline in quality of life. Brain-computer interfaces (BCIs) have emerged as an alternative instrument for helping AD patients communicate using brain signals to control external devices (Cassani et al., 2017). Based on Wolpaw (2013), conventional BCIs that rely on voluntary motor control may not be suitable for AD patients, who may have difficulty generating reliable motor commands.
A study by Liberati et al. (2012) has explored the use of classical conditioning and brain-state classification in combination to develop BCIs specifically tailored to AD patients’ needs. One study used classical conditioning to elicit a P300 ERP in response to a specific visual stimulus, which was then classified using a machine learning algorithm directing an electronic screen’s pointer (Haider & Fazel-Rezai, 2017). For instance, each column and row in the typing grid using the P300 speller are accelerated in an arbitrary sequence, with the entire six rows and columns accelerated in a single attempt (Manyakov et al., 2011). The study found that AD patients could control the pointer with the aid of the BCI, suggesting that classical conditioning can be an effective technique for eliciting reliable brain responses in AD patients. A different study by Heilinger et al. (2018) used a similar approach. However, instead of using a visual stimulus, the researchers used a vibrotactile stimulus applied to the wrist to elicit a P300 ERP. The study found that AD patients could learn to control a robotic arm using the BCI, indicating that the technique may help develop BCIs that allow AD patients to control external devices without relying on voluntary motor control.
Limitations and Future Directions
Several issues need to be resolved even though the combination of classical training and brain-state categorization showed potential for creating BCIs for AD patients. One drawback is that utilizing classical conditioning requires significant training, which may be challenging for AD patients with poor learning and memory (Lemieux & Woodruff-Pak, 2007). Not all AD patients may also benefit from vibrotactile stimuli because some may have reduced touch perception (Campbell et al., 2022). Future research should focus on developing more efficient and practical techniques for eliciting reliable brain responses in AD patients, such as using auditory or olfactory stimuli (Romano et al., 2020). Additionally, Kawala-Sterniuk et al. (2021); Simon et al. (2021) suggest that researchers should explore the potential for combining classical conditioning and brain-state classification with other BCI techniques, such as passive or active motor imagery, to develop BCIs that are tailored to the specific needs of individual AD patients.
METHODOLOGY
The initial and essential steps to comprehend the extent to which BCI can be utilized to support learning while sleeping are in understanding the historical context of its premise and elaborating on the variations and similarities in the publications of various scholars in academic circles. The academic literature discusses multiple concepts, guidelines, strategies, and components with the development of BCI technology and its integration into user-system communication. An extensive evaluation of databases and other resources was undertaken to retrieve relevant studies that were subsequently scrutinized and selected under the predetermined inclusion and exclusion requirements. This selection method was employed to guarantee the study’s authenticity and accuracy. The secondary objectives were identifying inadequacies in the body of literature and suggesting proposals for additional research.
Search Strategy
Different scholarly databases, including PubMed(NCBI), MPDI, ResearchGate, SAGE Journals, ScienceDirect, Google Scholar, and Frontiers, were accessed for an extensive and current literature evaluation. The phrases “brain-computer interface” (BCI), “sleep,” “learning,” “learning during sleep,” “memory consolidation,” “neurofeedback during sleep,” “memory consolidation during sleep, “BCI and sleep,” “neurofeedback,” “EEG,” and “neural plasticity” were all combined in the quest for information. Articles released in English-language periodicals between 2000 and 2023 were the sole sources included in the analysis. The search procedure was further narrowed by reviewing the reference lists of relevant articles, assessments, and systematic reviews to uncover additional literature that suited the criteria for acceptance.
Inclusion and Exclusion Grounds
The study considered only peer-reviewed research articles that examined the use of BCI to improve learning while asleep. Studies that used intrusive techniques, such as electrical brain stimulation, were excluded. The studies had to incorporate objective learning measures, such as identification or memory tests, and demonstrate statistically significant results. Studies that failed to indicate their findings were also disregarded. Reviews and meta-analyses were omitted, and the review only considered research that presented original data. Additionally, studies that were not published in English-language publications or those that were published earlier than January 2000 were disregarded.
Selection Procedure
The original search resulted in 250 publications following the selection standards applied. The titles and abstracts were examined to evaluate the publications’ relevance. The sources that satisfied the requirements for inclusion were then selected for a full-text examination. A total of 100 articles were chosen for full-text evaluation. The full-text assessment considered the quality of the studies and the practical relevance of the topic under study within the publications. Seventy-five papers were incorporated in the ultimate review after publications that failed to meet the inclusion parameters or had poor research quality were eliminated.
The key concepts that emerged after elimination parameters were determined using a meta-analysis, systematic assessments, and original research. The research selected for a review focused on the possibility that BCI could improve learning, memory consolidation, and cognitive function while sleeping. Studies that should have investigated using BCI for sleep-related learning mainly should have been addressed. A reference analysis was done to pinpoint notable scholars in the discipline. Authors of publications with many citations were identified, and the material of their published work was evaluated for relevance to the subject.
Screening and Evaluation
After removing redundant articles, publications were scrutinized by reviewing their headings to ensure they met the examination’s objectives and inclusion or exclusion parameters. After the initial review, the complete texts of every article were reviewed, and their suitability was determined following the preceding requirements. Thematic analysis was used to examine the data obtained from the selected research. The main subjects covered the possibility of using BCI to improve learning while sleeping, mental agility, and retention of information. Technical constraints, ethical issues, and prospective detrimental impacts on sleep quality were also noted as potential roadblocks to using brain-computer interface (BCI) technology to acquire knowledge during sleep.
Ethical Issues
Since the study utilized evidence easily obtainable from published research and never collected any original data, ethical concerns proved irrelevant.
Results
A collection of 492 entries were found in the initial phase of browsing, and they included the following: ResearchGate (109 results), Frontiers (89), SAGE Journals (24), ScienceDirect (56), MPDI (18), PubMed (73), and Google Scholar (123). After that, 168 matches were found when repetitions were automatically removed. In addition, 112 publications emerged in the initial abstract and title assessment, and 77 were added to the final repository following full-text analysis. During the full-text reading, publications were dismissed since they constituted instructive documents without peer review and needed to align with the topic under review.
ANALYSIS
An emerging field of study explores how Brain-Computer Interfaces (BCI) can support learning during sleep. Based on this technique, BCI technology could promote memory consolidation and enhance learning by selectively engaging specific brain regions while asleep. The critically obtained knowledge is consolidated into preexisting memory structures through a process known as memory consolidation. The entire process is supposed to take place during sleep, where the brain can enhance memories by playing them back. Memory consolidation during sleep is facilitated by using non-surgical techniques such as transcranial magnetic stimulation or transcranial direct current stimulating (tDCS), as shown in numerous research (Prehn-Kristensen et al., 2014). Brain stimulation (BCI) offers an effective way to stimulate specific brain regions when sleeping selectively. BCI systems use EEG or fMRI signals to assess brain activity connected to certain mental states or tasks. These networks then employ this information to control extraneous devices or provide a response to the user. Numerous studies (Born & Wilhelm, 2011); (Stickgold & Walker, 2007) have demonstrated the potential of using BCI technology to selectively engage certain brain areas connected to memory consolidation while a person is sleeping.
Significant discrepancies in knowledge exist concerning the application of BCI to support learning during sleep, notwithstanding the positive findings to date. There need to be more samples, and the consistency across ethnicities are two major drawbacks of current research. Most research has been done on healthy adolescents and young adults; thus, it is not apparent if the findings apply to other groups, such as elderly individuals or those experiencing sleep difficulties. In addition, more research is required to determine the long-term impact of BCI-based therapies on memory consolidation while we sleep. The lack of uniformity in the techniques employed to identify and categorize various sleep phases is another limitation of previous research. It is challenging to compare the findings of multiple investigations because they have used distinct norms and methodologies to categorize the various stages of sleep. Procedures and standards for sleep grading need to be standardized to guarantee the validity and dependability of BCI-based solutions to enhance learning during sleep,
Addressing these gaps requires a transdisciplinary strategy for investigating BCI-based learning therapies. Scholars from various disciplines, including medical science, engineering, mental health, and neuroscience, would collaborate in such a strategy. By merging knowledge from many fields of study, we can build more comprehensive and all-encompassing techniques to look into the possible uses of BCI in this subject. For instance, a partnership involving engineers and neuroscientists could create more advanced BCI systems that can more accurately and consistently detect and activate specific brain regions. The partnership amongst experts in psychology and medicine may be able to pinpoint the most successful interventions for various groups depending on individual variations in sleep habits and cognitive capacities.
References
Abdulkader, S. N., Atia, A., & Mostafa, M.-S. M. (2015). Brain computer interfacing: Applications and challenges. Egyptian Informatics Journal, 16(2), 213–230. https://doi.org/10.1016/j.eij.2015.06.002
Alger, S., Chambers, A., Cunningham, T. J., & Payne, J. D. (2014, September 17). The Role of Sleep in Human Declarative Memory Consolidation. ResearchGate; unknown.
Andrillon, T., & Kouider, S. (2016). Implicit memory for words heard during sleep. ResearchGate; Oxford University Press. https://www.researchgate.net/publication/306927826_Implicit_memory_for_words_heard_during_sleep
Axelsson, E. L., Williams, S. E., & Horst, J. S. (2016). The Effect of Sleep on Children’s Word Retention and Generalization. Frontiers in Psychology, 7. https://doi.org/10.3389/fpsyg.2016.01192
Batterink, L., Creery, J., & Paller, K. (2016, January 27). Phase of Spontaneous Slow Oscillations during Sleep Influences Memory-Related Processing of Auditory Cues. ResearchGate; Society for Neuroscience. https://www.researchgate.net/publication/292178090_Phase_of_Spontaneous_Slow_Oscillations_during_Sleep_Influences_Memory-Related_Processing_of_Auditory_Cues
Born, J., & Wilhelm, I. (2011). System consolidation of memory during sleep. Psychological Research, 76(2), 192–203. https://doi.org/10.1007/s00426-011-0335-6
Born, J., & Wilhelm, I. (2011). System consolidation of memory during sleep. Psychological Research, 76(2), 192–203. https://doi.org/10.1007/s00426-011-0335-6
Brito, D. (2021, February 19). Molecular Mechanisms of Memory Formation and Persistence in the Adult and Aged Brain. ResearchGate; unknown. https://www.researchgate.net/publication/349963812_Molecular_Mechanisms_of_Memory_Formation_and_Persistence_in_the_Adult_and_Aged_Brain
Camargo-Vargas, D., Callejas-Cuervo, M. and Mazzoleni, S. (2021). Brain-Computer Interfaces Systems for Upper and Lower Limb Rehabilitation: A Systematic Review. Sensors, [online] 21(13), p.4312. doi:https://doi.org/10.3390/s21134312.
Campbell, E. A., Kantor, J., Kantorová, L., Svobodová, Z., & Wosch, T. (2022). Tactile Low Frequency Vibration in Dementia Management: A Scoping Review. Frontiers in Psychology, 13. https://doi.org/10.3389/fpsyg.2022.854794
Cao, Z. (2020). A review of artificial intelligence for EEG‐based brain−computer interfaces and applications – Zehong Cao, 2020. [online] Brain Science Advances. Available at: https://journals.sagepub.com/doi/full/10.26599/BSA.2020.9050017
Chaudhary, U., Vlachos, I., Zimmermann, J. B., Espinosa, A., Tonin, A., Jaramillo-Gonzalez, A., Khalili-Ardali, M., Topka, H., Lehmberg, J., Friehs, G. M., Woodtli, A., Donoghue, J. P., & Birbaumer, N. (2022). Spelling interface using intracortical signals in a completely locked-in patient enabled via auditory neurofeedback training. Nature Communications, 13(1). https://doi.org/10.1038/s41467-022-28859-8
Chikara, R. K., Lo, W.-C., & Ko, L.-W. (2020). Exploration of Brain Connectivity during Human Inhibitory Control Using Inter-Trial Coherence. Sensors, 20(6), 1722. https://doi.org/10.3390/s20061722
Colten, H. R., Altevogt, B. M., & US), M. (2023). Sleep Physiology. Nih.gov; National Academies Press (US). https://www.ncbi.nlm.nih.gov/books/NBK19956/
Cousins, J., & Guillén Fernández. (2019). The impact of sleep deprivation on declarative memory. ResearchGate; Elsevier. https://www.researchgate.net/publication/331945659_The_impact_of_sleep_deprivation_on_declarative_memory
Cristiane Furini, Nachtigall, E., Kielbovicz, A., & Izquierdo, I. (2020, April). Molecular Mechanisms in Hippocampus Involved on Object Recognition Memory Consolidation and Reconsolidation. ResearchGate; Elsevier. https://www.researchgate.net/publication/340471863_Molecular_Mechanisms_in_Hippocampus_Involved_on_Object_Recognition_Memory_Consolidation_and_Reconsolidation
Cunningham, T. J., & Payne, J. D. (2017, February 10). Emotional Memory Consolidation During Sleep. ResearchGate; unknown. https://www.researchgate.net/publication/313541905_Emotional_Memory_Consolidation_During_Sleep
Cunningham, T. J., Enmanuelle Pardilla-Delgado, Alger, S., & Payne, J. D. (2014, August 8). The Role of REM Sleep in Emotional Memory and Affective Reactivity in Humans. ResearchGate; unknown. https://www.researchgate.net/publication/267508090_The_Role_of_REM_Sleep_in_Emotional_Memory_and_Affective_Reactivity_in_Humans
Cassani, R., Falk, T. H., Fraga, F. J., & Anghinah, R. (2017, March). Towards automated electroencephalography-based Alzheimer’s disease diagnosis using portable low-density… ResearchGate; Elsevier. https://www.researchgate.net/publication/311824171_Towards_automated_electroencephalography-based_Alzheimer’s_disease_diagnosis_using_portable_low-density_devices
Christoforou, C. (2017). Classical Conditioning. Encyclopedia of Evolutionary Psychological Science, 1–10. https://doi.org/10.1007/978-3-319-16999-6_1039-1
Dheeraj Rathee, Raza, H., Roy, S., & Prasad, G. (2021, April 29). Magnetoencephalography (MEG)-based Brain-Computer Interface for detecting Motor and Cognitive Imagery. ResearchGate; Springer Nature. https://www.researchgate.net/publication/351118407_Magnetoencephalography_MEG-based_Brain-Computer_Interface_for_detecting_Motor_and_Cognitive_Imagery
Deldar, Z., Gevers-Montoro, C., Khatibi, A., & Ghazi-Saidi, L. (2020). The interaction between language and working memory: a systematic review of fMRI studies in the past two decades. AIMS Neuroscience, 8(1), 1–32. https://doi.org/10.3934/neuroscience.2021001
Dudai, Y., Karni, A., & Born, J. (2015). The Consolidation and Transformation of Memory. Neuron, 88(1), 20–32. https://doi.org/10.1016/j.neuron.2015.09.004
Edlow, B. L., Claassen, J., Schiff, N. D., & Greer, D. M. (2020). Recovery from disorders of consciousness: mechanisms, prognosis and emerging therapies. Nature Reviews Neurology, 17(3), 135–156. https://doi.org/10.1038/s41582-020-00428-x
Fred, A.L., Kumar, S.N., Kumar Haridhas, A., Ghosh, S., Purushothaman Bhuvana, H., Sim, W.K.J., Vimalan, V., Givo, F.A.S., Jousmäki, V., Padmanabhan, P. and Gulyás, B. (2022). A Brief Introduction to Magnetoencephalography (MEG) and Its Clinical Applications. Brain Sciences, 12(6), p.788. doi:https://doi.org/10.3390/brainsci12060788.
Feld, G. B., Weis, P. P., & Born, J. (2016). The Limited Capacity of Sleep-Dependent Memory Consolidation. Frontiers in Psychology, 7. https://doi.org/10.3389/fpsyg.2016.01368
Flesher, S. N., Downey, J. E., Weiss, J. M., Hughes, C. L., Herrera, A. J., Tyler-Kabara, E. C., Boninger, M. L., Collinger, J. L., & Gaunt, R. A. (2021). A brain-computer interface that evokes tactile sensations improves robotic arm control. Science, 372(6544), 831–836. https://doi.org/10.1126/science.abd0380
Gibson, R. M., Owen, A. M., & Cruse, D. (2016). Brain–computer interfaces for patients with disorders of consciousness. Progress in Brain Research, 241–291. https://doi.org/10.1016/bs.pbr.2016.04.003
Guo, M., Moroke Igue, Malhotra, A., & Djonlagic, I. (2013, December). The effect of obstructive sleep apnea on declarative memory consolidation. ResearchGate; Elsevier. https://www.researchgate.net/publication/275319120_The_effect_of_obstructive_sleep_apnea_on_declarative_memory_consolidation
Hall, N. J., Smith, D. W., & Wynne, C. D. L. (2015). Pavlovian conditioning enhances resistance to disruption of dogs performing an odor discrimination. Journal of the Experimental Analysis of Behavior, 103(3), 484–497. https://doi.org/10.1002/jeab.151
Heilinger, A., Ortner, R., La Bella, V., Lugo, Z. R., Chatelle, C., Laureys, S., Spataro, R., & Guger, C. (2018). Performance Differences Using a Vibro-Tactile P300 BCI in LIS-Patients Diagnosed With Stroke and ALS. Frontiers in Neuroscience, 12. https://doi.org/10.3389/fnins.2018.00514
https://www.researchgate.net/publication/265791872_The_Role_of_Sleep_in_Human_Declarative_Memory_Consolidation
Ilyas, M. Z., Saad, P., Ahmad, M. I., & Ghani, I. (2016, November). Classification of EEG signals for brain-computer interface applications: Performance comparison. ResearchGate; unknown. https://www.researchgate.net/publication/314667794_Classification_of_EEG_signals_for_brain-computer_interface_applications_Performance_comparison
Jamil, N., Abdelkader Nasreddine Belkacem, Ouhbi, S., & Christoph Guger. (2021, September 23). Cognitive and Affective Brain–Computer Interfaces for Improving Learning Strategies and Enhancing Student… ResearchGate; unknown. https://www.researchgate.net/publication/354795317_Cognitive_and_Affective_Brain-Computer_Interfaces_for_Improving_Learning_Strategies_and_Enhancing_Student_Capabilities_A_Systematic_Literature_Review
Kawala-Sterniuk, A., Browarska, N., Al-Bakri, A., Pelc, M., Zygarlicki, J., Sidikova, M., Martinek, R., & Gorzelanczyk, E. J. (2021). Summary of over Fifty Years with Brain-Computer Interfaces—A Review. Brain Sciences, 11(1), 43. https://doi.org/10.3390/brainsci11010043
Kumar, A., Sidhu, J., Goyal, A., & Tsao, J. W. (2022, June 5). Alzheimer Disease. Nih.gov; StatPearls Publishing. https://www.ncbi.nlm.nih.gov/books/NBK499922/
Lemieux, S., & Woodruff-Pak, D. S. (2007, May 8). Functional MRI Studies of Eyeblink Classical Conditioning. ResearchGate; unknown. https://www.researchgate.net/publication/226554480_Functional_MRI_Studies_of_Eyeblink_Classical_Conditioning
Liberati, G., Dalboni, J., Linda, & Sitaram, R. (2012, March 26). Toward a Brain-Computer Interface for Alzheimer’s Disease Patients by Combining Classical Conditioning and… ResearchGate; IOS Press. https://www.researchgate.net/publication/221982782_Toward_a_Brain-Computer_Interface_for_Alzheimer’s_Disease_Patients_by_Combining_Classical_Conditioning_and_Brain_State_Classification
Mikołajewska, E., & Mikołajewski, D. (2014). Non-invasive EEG-based brain-computer interfaces in patients with disorders of consciousness. Military Medical Research, 1(1), 14. https://doi.org/10.1186/2054-9369-1-14
Manyakov, N.V., Chumerin, N., Combaz, A. and Van Hulle, MM (2011). Comparison of Classification Methods for P300 Brain-Computer Interface on Disabled Subjects. Computational Intelligence and Neuroscience, [online] 2011, pp.1–12. doi:https://doi.org/10.1155/2011/519868.
Maquet, P. (2001). The Role of Sleep in Learning and Memory. Science, 294(5544), 1048–1052. https://doi.org/10.1126/science.1062856
Md Ali Haider, & Reza Fazel-Rezai. (2017, November 29). Application of P300 Event-Related Potential in Brain-Computer Interface. ResearchGate; unknown. https://www.researchgate.net/publication/321394222_Application_of_P300_Event-Related_Potential_in_Brain-Computer_Interface
Mudgal, S. K., Sharma, S. K., Chaturvedi, J., & Sharma, A. (2020). Brain computer interface advancement in neurosciences: Applications and issues. Interdisciplinary Neurosurgery, 20, 100694. https://doi.org/10.1016/j.inat.2020.100694
Navarrete, M. and Lewis, P.A. (2019). Cognition: Learning while Asleep. Current Biology, [online] 29(5), pp.R164–R166. doi:https://doi.org/10.1016/j.cub.2019.01.034.
Navarrete, M., & Lewis, P. A. (2019). Cognition: Learning while Asleep. Current Biology, 29(5), R164–R166. https://doi.org/10.1016/j.cub.2019.01.034
Nemeth, D., Gerbier, E., Born, J., & Karolina Janacsek. (2021, December 13). Pitfalls in Sleep and Memory Research and How to Avoid Them: A Consensus Paper. ResearchGate; unknown. https://www.researchgate.net/publication/357041778_Pitfalls_in_Sleep_and_Memory_Research_and_How_to_Avoid_Them_A_Consensus_Paper
Neumann, F., Oberhauser, V., & Kornmeier, J. (2020). How odor cues help to optimise learning during sleep in a real life-setting. Scientific Reports, 10(1). https://doi.org/10.1038/s41598-020-57613-7
Paller, K. A., & Voss, J. L. (2004). Memory reactivation and consolidation during sleep. Learning & Memory, 11(6), 664–670. https://doi.org/10.1101/lm.75704
Payne, J. D., Chambers, A. M., & Kensinger, E. A. (2012). Sleep promotes lasting changes in selective memory for emotional scenes. Frontiers in Integrative Neuroscience, p. 6. https://doi.org/10.3389/fnint.2012.00108
Pearson, H., & Wilbiks, J. (2021). Effects of Audiovisual Memory Cues on Working Memory Recall. Vision, 5(1), 14. https://doi.org/10.3390/vision5010014
Poe, G. R., Walsh, C. M., & Bjorness, T. E. (2010). Cognitive neuroscience of sleep. Progress in Brain Research, pp. 1–19. https://doi.org/10.1016/b978-0-444-53702-7.00001-4
Prehn-Kristensen, A., Munz, M., Göder, R., Wilhelm, I., Korr, K., Vahl, W., Wiesner, C. D., & Baving, L. (2014). Transcranial Oscillatory Direct Current Stimulation During Sleep Improves Declarative Memory Consolidation in Children With Attention-deficit/hyperactivity Disorder to a Level Comparable to Healthy Controls. Brain Stimulation, 7(6), 793–799. https://doi.org/10.1016/j.brs.2014.07.036
Puchkova, A. N. (2020). Studies of Learning during Sleep: Problems, Progress, and Perspectives. Neuroscience and Behavioral Physiology, 50(3), 257–263. https://doi.org/10.1007/s11055-020-00895-1
Park, K. S. (2023). Brain-Computer Interface. Humans and Electricity, 223–248. https://doi.org/10.1007/978-3-031-20784-6_10
Poe, G. R., Walsh, C. M., & Bjorness, T. E. (2010). Both Duration and Timing of Sleep are Important to Memory Consolidation. Sleep, 33(10), 1277–1278. https://doi.org/10.1093/sleep/33.10.1277
Porsteinsson, A. P., Isaacson, R. S., Knox, S., Sabbagh, M. N., & Rubino, I. (2021). Diagnosis of Early Alzheimer’s Disease: Clinical Practice in 2021. The Journal of Prevention of Alzheimer’s Disease, 1–16. https://doi.org/10.14283/jpad.2021.23
Reyes-Resina, I., Samer, S., Kreutz, M. R., & Oelschlegel, A. M. (2021). Molecular Mechanisms of Memory Consolidation That Operate During Sleep. Frontiers in Molecular Neuroscience, 14. https://doi.org/10.3389/fnmol.2021.767384
Ruch, S., Alain Züst, M., & Henke, K. (2022). Sleep-learning impairs subsequent awake-learning. Neurobiology of Learning and Memory, 187, 107569. https://doi.org/10.1016/j.nlm.2021.107569
Ruf, C. A., De Massari, D., Furdea, A., Matuz, T., Fioravanti, C., van der Heiden, L., Halder, S., & Birbaumer, N. (2013). Semantic Classical Conditioning and Brain-Computer Interface Control: Encoding of Affirmative and Negative Thinking. Frontiers in Neuroscience, 7. https://doi.org/10.3389/fnins.2013.00023
Rasch, B., & Born, J. (2013). About Sleep’s Role in Memory. Physiological Reviews, 93(2), 681–766. https://doi.org/10.1152/physrev.00032.2012
Reyes-Resina, I., Samer, S., Kreutz, M. R., & Oelschlegel, A. M. (2021). Molecular Mechanisms of Memory Consolidation That Operate During Sleep. Frontiers in Molecular Neuroscience, 14. https://doi.org/10.3389/fnmol.2021.767384
Romano, R. R., Carter, M. A., & Monroe, T. B. (2020). Narrative Review of Sensory Changes as a Biomarker for Alzheimer’s Disease. Biological Research for Nursing, 23(2), 223–230. https://doi.org/10.1177/1099800420947176
Ruch, S., & Henke, K. (2020, January 15). Learning During Sleep: A Dream Comes True? ResearchGate; Elsevier. https://www.researchgate.net/publication/338615751_Learning_During_Sleep_A_Dream_Comes_True
Schechtman, E., Heilberg, J., & Paller, K. A. (2023). Memory consolidation during sleep involves context reinstatement in humans. Cell Reports, 42(4), 112331. https://doi.org/10.1016/j.celrep.2023.112331
Sevasti Kapsi, Spiridoula Katsantoni, & Athanasios Drigas. (2020, September 30). The Role of Sleep and Impact on Brain and Learning. ResearchGate; International Association of Online Engineering. https://www.researchgate.net/publication/344433014_The_Role_of_Sleep_and_Impact_on_Brain_and_Learning
Simon, C., Bolton, D. A. E., Kennedy, N. C., Soekadar, S. R., & Ruddy, K. L. (2021). Challenges and Opportunities for the Future of Brain-Computer Interface in Neurorehabilitation. Frontiers in Neuroscience, 15. https://doi.org/10.3389/fnins.2021.699428
Spataro, R., Xu, Y., Xu, R., Mandalà, G., Allison, B. Z., Ortner, R., Heilinger, A., La Bella, V., & Guger, C. (2022). How brain-computer interface technology may improve the diagnosis of the disorders of consciousness: A comparative study. Frontiers in Neuroscience, 16. https://doi.org/10.3389/fnins.2022.959339
Schimke, E. A. E., Angwin, A. J., Cheng, B. B. Y., & Copland, D. A. (2021). The effect of sleep on novel word learning in healthy adults: A systematic review and meta-analysis. Psychonomic Bulletin & Review, 28(6), 1811–1838. https://doi.org/10.3758/s13423-021-01980-3
Stickgold, R., & Walker, M. (2007, July). Sleep-Dependent Memory Consolidation and Reconsolidation. ResearchGate; Elsevier. https://www.researchgate.net/publication/6360452_Sleep-Dependent_Memory_Consolidation_and_Reconsolidation
Tamminen, J., Newbury, C. R., Crowley, R., Vinals, L., Cevoli, B., & Rastle, K. (2020). Generalisation in language learning can withstand total sleep deprivation. Neurobiology of Learning and Memory, 173, 107274. https://doi.org/10.1016/j.nlm.2020.107274
Valeriani, D., Santoro, F., & Ienca, M. (2022). The present and future of neural interfaces. Frontiers in Neurorobotics, 16. https://doi.org/10.3389/fnbot.2022.953968
van, & Walker, M. (2011, March). Sleep and Emotional Memory Processing. ResearchGate; WB Saunders. https://www.researchgate.net/publication/251690128_Sleep_and_Emotional_Memory_Processing
Vulić, K., Bjekić, J., Paunović, D., Jovanović, M., Milanović, S., & Filipović, S. R. (2021). Theta-modulated oscillatory transcranial direct current stimulation over the posterior parietal cortex improves associative memory. Scientific Reports, 11(1). https://doi.org/10.1038/s41598-021-82577-7
Wang, F. (2020, January 2). Sleep and Memory Consolidation. ResearchGate; unknown. https://www.researchgate.net/publication/338377309_Sleep_and_Memory_Consolidation
Wang, H., Xu, J., Lazarovici, P., Quirion, R., & Zheng, W. (2018). cAMP Response Element-Binding Protein (CREB): A Possible Signaling Molecule Link in the Pathophysiology of Schizophrenia. Frontiers in Molecular Neuroscience, 11. https://doi.org/10.3389/fnmol.2018.00255
Wolpaw, J. R. (2013). Brain–computer interfaces. Neurological Rehabilitation, 67–74. https://doi.org/10.1016/b978-0-444-52901-5.00006-x
Zhu, Y., Wang, Q., & Zhang, L. (2021). Study of EEG characteristics while solving scientific problems with different mental effort. Scientific Reports, 11(1). https://doi.org/10.1038/s41598-021-03321-9